Google Vs. OpenAI: A Deep Dive Into I/O And Io Technologies
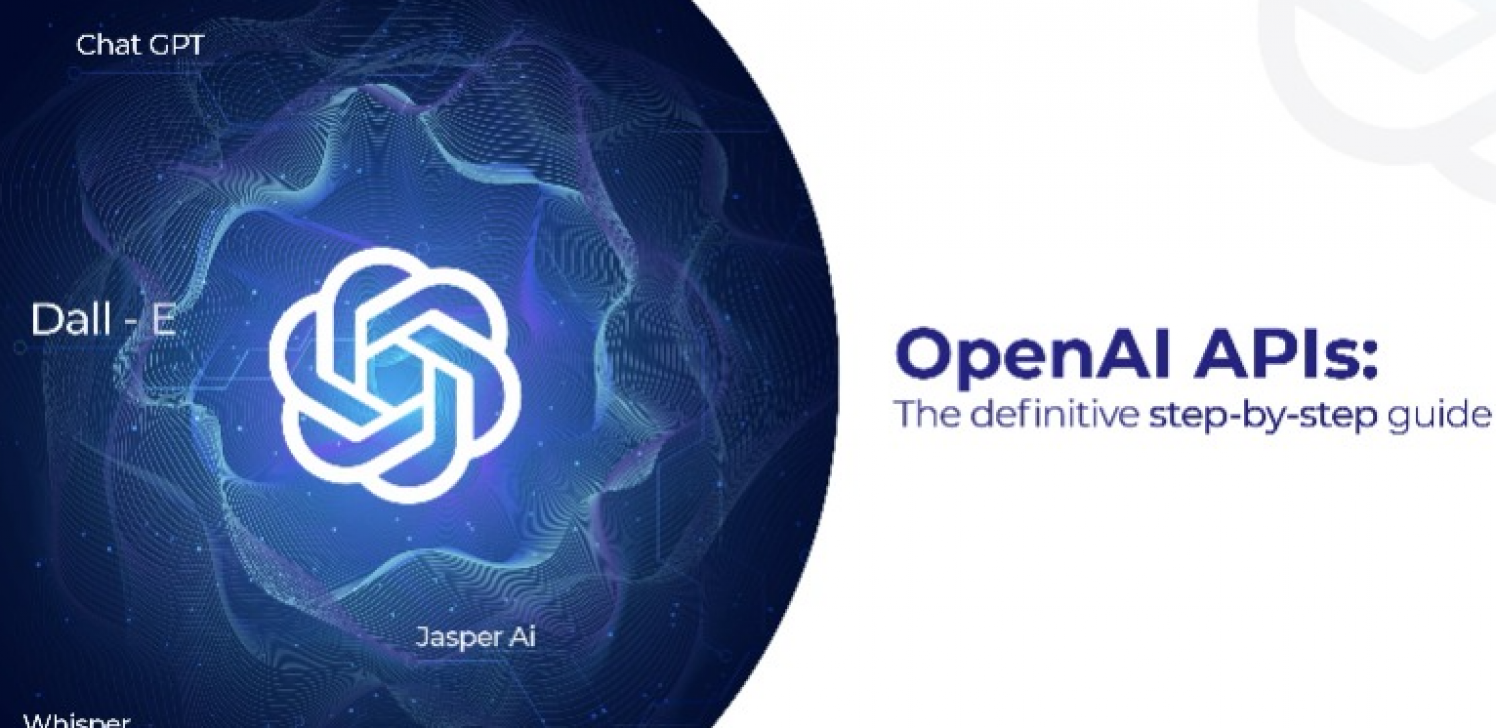
Table of Contents
Google's I/O and io Technologies: A Focus on Scalability and Infrastructure
Google's dominance in AI is deeply rooted in its massive infrastructure and its commitment to scalability. This section examines the key I/O technologies underpinning Google's AI achievements.
Google's Cloud Platform (GCP) and its Role in AI Development
Google Cloud Platform (GCP) serves as the bedrock for Google's AI development. Its vast infrastructure allows for the training and deployment of massive AI models demanding immense computational power and data throughput.
- Massive Scalability: GCP's infrastructure can handle petabytes of data and thousands of concurrent tasks, crucial for training large language models and other complex AI systems. This scalability directly addresses the I/O challenges posed by the sheer volume of data involved in AI.
- Specialized AI/ML Tools: TensorFlow, a leading machine learning framework, and Vertex AI, a managed machine learning service, are integral parts of GCP, streamlining the development and deployment process and optimizing I/O operations.
- TPU Advantage: Google's Tensor Processing Units (TPUs) are custom-designed hardware accelerators specifically optimized for machine learning workloads. TPUs significantly enhance I/O performance by accelerating data processing and reducing latency, leading to faster model training and inference. This provides a significant advantage in handling the high I/O demands of modern AI models.
- Efficient Data Storage: GCP offers a range of storage solutions, from high-performance SSDs to cost-effective cloud storage, ensuring efficient data ingestion and retrieval, crucial aspects of optimized I/O.
Google's infrastructure supports the massive I/O demands of large language models like LaMDA and PaLM through sophisticated data sharding and parallel processing techniques. The integration of TPUs further accelerates the process, minimizing I/O bottlenecks.
Google's Approach to Input/Output (I/O) Optimization
Google employs a multi-pronged strategy to optimize I/O in its AI systems. This approach goes beyond individual components and focuses on holistic system optimization.
- Data Parallelism: Distributing data across multiple processors allows for parallel processing, drastically reducing the time needed for training and inference. This is critical for handling the large datasets common in AI.
- Distributed Computing: Google leverages its vast network of data centers and its expertise in distributed systems to handle complex computations efficiently, optimizing data flow and minimizing I/O latency.
- Efficient Data Storage and Retrieval: Strategic placement of data across the GCP network, coupled with sophisticated caching mechanisms, ensures rapid access to data, reducing I/O wait times and improving overall efficiency. This minimizes bottlenecks in data access.
- Custom Hardware and Software Co-design: Google's integrated approach, combining custom hardware (TPUs) with optimized software, leads to synergistic improvements in I/O performance.
These techniques, working together, allow Google to achieve unparalleled performance in training and deploying massive AI models, demonstrating a mastery of high-throughput I/O management.
OpenAI's I/O and io Technologies: Prioritizing Model Performance and Efficiency
OpenAI takes a different approach, prioritizing model performance and developer accessibility. Its focus is on creating efficient models, rather than solely building massive infrastructure.
OpenAI's API and its Ecosystem
OpenAI's primary interface for accessing its models is through its API. This provides a convenient and accessible way for developers to integrate powerful AI capabilities into their applications.
- API Accessibility: The OpenAI API simplifies the process of interacting with models like GPT-3 and DALL-E, abstracting away much of the underlying infrastructure complexity. This accessibility is a key differentiator from Google's infrastructure-focused approach.
- Model Diversity: OpenAI offers a range of models, each optimized for different tasks, catering to various I/O needs. The selection of the appropriate model helps manage I/O efficiently for specific applications.
- Developer Ecosystem: A thriving community of developers utilizes the OpenAI API, constantly pushing the boundaries of what's possible and providing valuable feedback that improves model performance and I/O efficiency.
This API-centric approach allows OpenAI to focus on model optimization, leaving much of the I/O management to its users or its underlying infrastructure providers.
OpenAI's Focus on Model Optimization for Efficient I/O
OpenAI employs sophisticated techniques to create computationally efficient models that minimize I/O bottlenecks. This allows for faster inference and reduced resource consumption.
- Quantization: Reducing the precision of model parameters lowers memory requirements and accelerates computation, directly improving I/O efficiency.
- Pruning: Removing less important connections in the neural network reduces model size and complexity, leading to faster processing and less I/O overhead.
- Knowledge Distillation: Training smaller, faster "student" models to mimic the behavior of larger, more complex "teacher" models significantly reduces the I/O demands of inference.
These model-centric optimization techniques directly impact I/O efficiency by reducing the computational load and data transfer requirements, making powerful AI accessible even on resource-constrained devices.
Comparing Google and OpenAI: A Head-to-Head Analysis of I/O Approaches
Google and OpenAI employ distinct strategies for I/O optimization, each with its own strengths and weaknesses.
Scalability vs. Accessibility:
Google prioritizes massive scalability, enabling the training of colossal models. OpenAI focuses on ease of access via its API, enabling broader adoption. Google's approach excels in computationally intensive tasks, while OpenAI's excels in accessibility and ease of integration.
Infrastructure vs. Model Optimization:
Google's approach is infrastructure-centric, leveraging its massive infrastructure to overcome I/O challenges. OpenAI's strategy is model-centric, optimizing models to minimize I/O bottlenecks. Google's strength lies in handling massive datasets, while OpenAI excels in creating efficient models that run on diverse platforms.
Future Implications and Trends:
Both companies will continue to innovate in I/O technologies. We can expect advancements in hardware acceleration, distributed computing, and model compression techniques. The future likely involves a hybrid approach, combining scalable infrastructure with highly optimized models.
Conclusion
This deep dive into Google and OpenAI's I/O and io technologies reveals distinct yet equally impressive strategies. Google prioritizes infrastructure scalability, while OpenAI emphasizes model optimization and developer accessibility. Both approaches are crucial to advancing the field of AI. Understanding the nuances of their respective I/O strategies is vital for developers, researchers, and anyone interested in the future of artificial intelligence. To stay informed about the latest advancements in Google and OpenAI’s I/O technologies and their impact on the AI landscape, continue exploring the latest research and developments in Google vs. OpenAI I/O and io technologies.
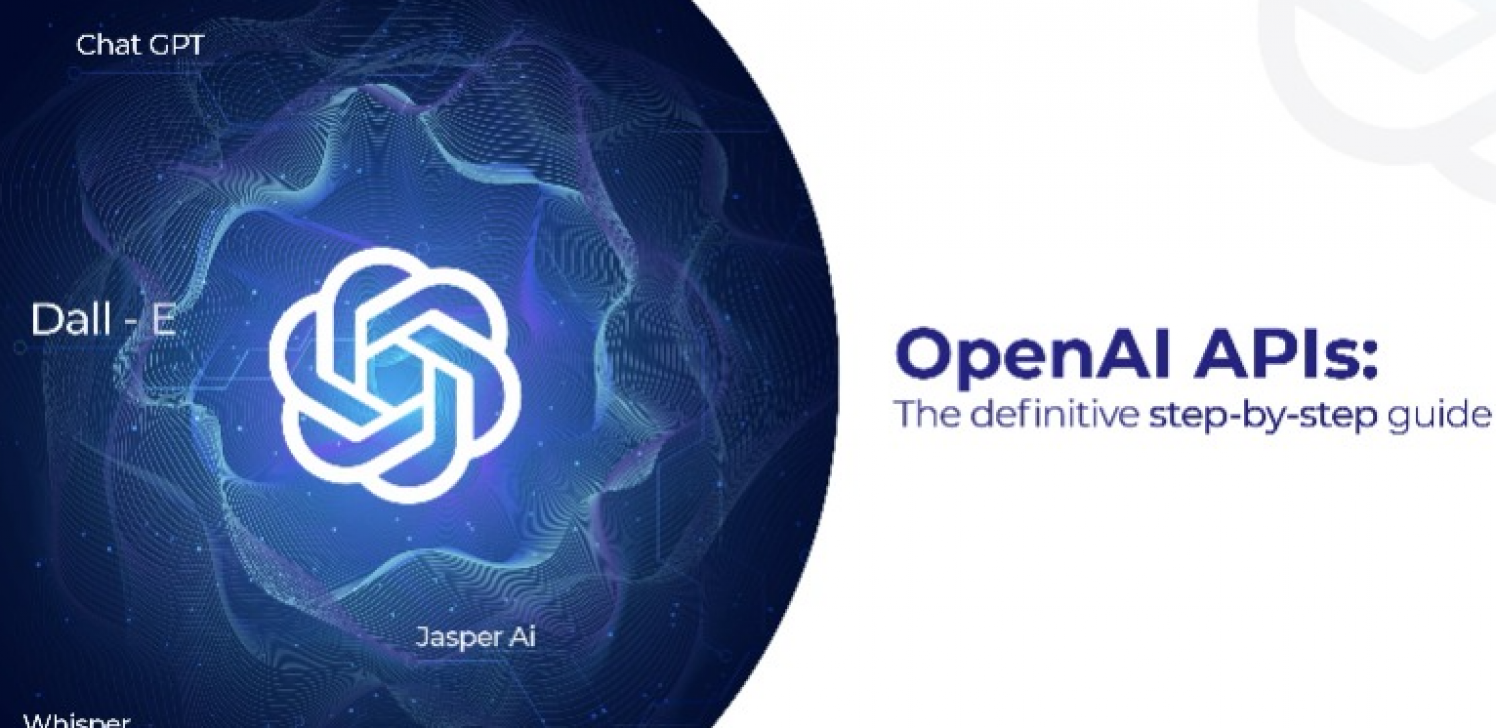
Featured Posts
-
Hoka Cielo X1 2 0 Running Shoe Review Is It Right For You
May 26, 2025 -
Canada Post Strike Will Customer Loyalty Suffer
May 26, 2025 -
Zize Humoriste Transformiste Spectacle 100 Marseillais A Graveson Le 4 Avril
May 26, 2025 -
Jadwal Dan Informasi Moto Gp Inggris 2025 Saksikan Aksi Para Pembalap
May 26, 2025 -
Dr Terrors House Of Horrors What To Expect On Your Visit
May 26, 2025