Outdated Business Apps: How They Obscure Your AI Vision
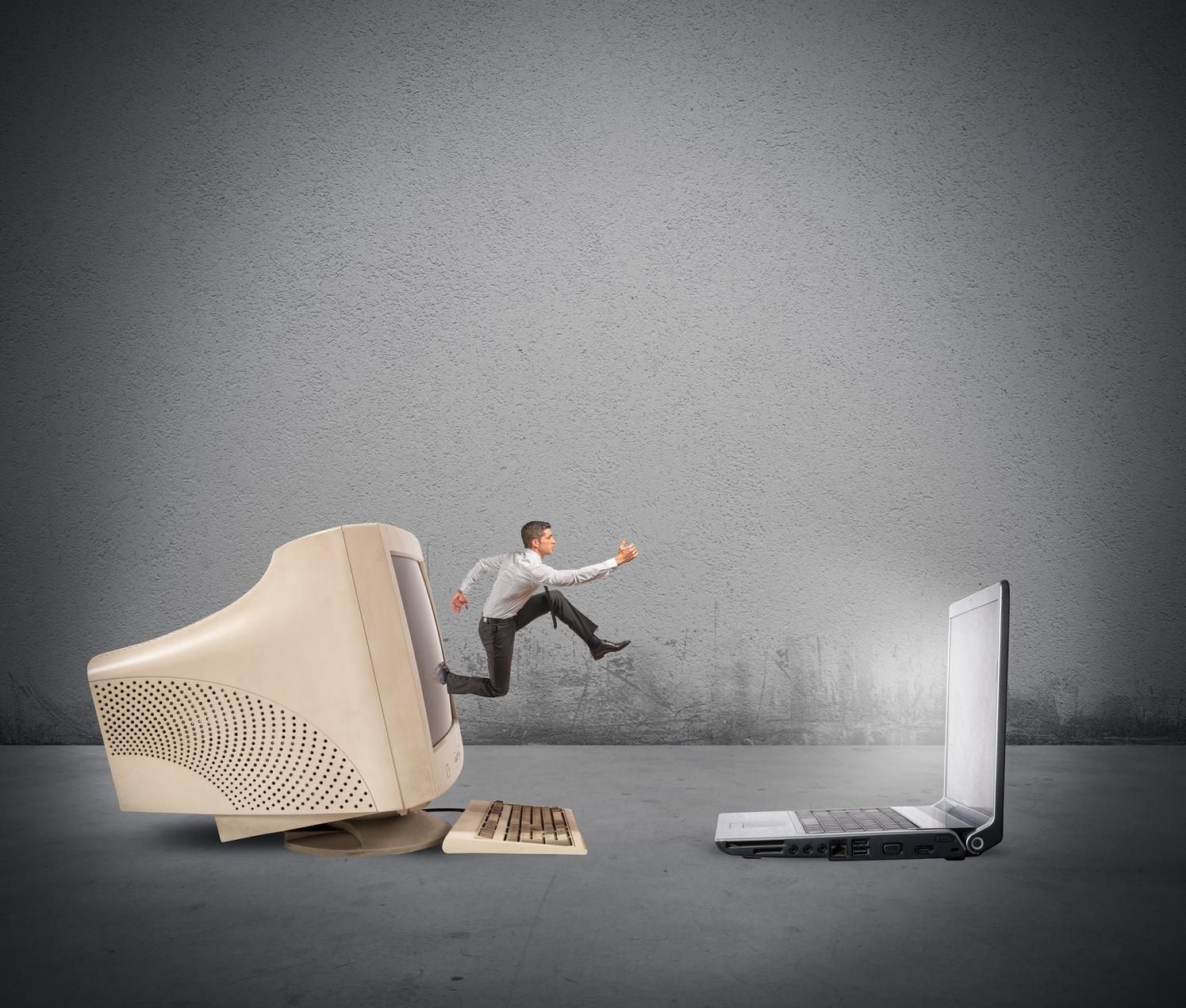
Table of Contents
Data Silos and Incompatibility: The Biggest Hurdles to AI Integration
Outdated business applications frequently present a major roadblock to effective AI integration due to data silos and format incompatibility. These issues significantly impact the ability of AI algorithms to access, process, and learn from the necessary data.
Data Isolation: The Silo Effect
Outdated apps often store data in isolated silos, hindering seamless access for AI algorithms. This data isolation creates significant challenges for machine learning.
- Lack of API integration: Many legacy systems lack the Application Programming Interfaces (APIs) needed for seamless data flow between different applications and AI platforms. This necessitates manual data extraction and transfer, a time-consuming and error-prone process.
- Difficulty in data extraction and transformation: Extracting data from outdated systems can be incredibly difficult. The data might be trapped in proprietary formats, requiring complex and costly data transformation processes before it can be used by AI systems.
- Increased risk of data inconsistency and errors: Manual data consolidation significantly increases the risk of data errors and inconsistencies. This can lead to inaccurate AI model training and unreliable results.
- Higher costs associated with manual data consolidation: The manual effort required to collect, clean, and prepare data from disparate sources adds considerable cost to AI implementation projects.
Format Incompatibility: A Tower of Babel for Data
Different legacy systems often use various data formats (e.g., CSV, XML, proprietary databases), creating further incompatibility challenges for AI platforms which often require specific data structures.
- Difficulty in converting data between formats: Converting data between incompatible formats is a complex and time-consuming task, prone to errors and potential data loss.
- Need for extensive data cleansing and preprocessing: Data from legacy systems often requires extensive cleaning and preprocessing before it's suitable for AI model training. This adds significant time and resources to the project.
- Increased development time and costs for AI integration: The complexities of data integration significantly increase development time and costs, delaying the realization of AI benefits.
- Potential for data loss or corruption during conversion: Data conversion processes, especially manual ones, carry the risk of data loss or corruption, impacting the accuracy and reliability of AI models.
Security Risks and Compliance Issues
Outdated business applications often pose significant security and compliance risks, creating substantial obstacles to successful AI deployment.
Vulnerability to Cyberattacks: A Legacy of Weakness
Legacy systems frequently lack the robust security features required to protect sensitive data used for AI, making them highly vulnerable to cyberattacks.
- Outdated security protocols and encryption methods: Many legacy systems employ outdated security protocols and encryption methods, making them easy targets for hackers.
- Lack of regular security updates and patching: Failure to regularly update and patch legacy systems leaves them vulnerable to known security vulnerabilities.
- Increased risk of data breaches and leaks: Outdated applications significantly increase the risk of data breaches and leaks, potentially exposing sensitive customer and business information.
- Non-compliance with data privacy regulations: Legacy systems may not comply with modern data privacy regulations (GDPR, CCPA, etc.), leading to hefty fines and legal repercussions.
Compliance Challenges: Meeting Regulatory Demands
Meeting industry regulations and compliance standards with legacy systems is extremely challenging. These systems often lack the auditing capabilities and data governance features crucial for AI deployment.
- Difficulty in tracking data lineage and provenance: Legacy systems may not provide adequate tools for tracking data lineage and provenance, making it difficult to demonstrate compliance.
- Challenges in demonstrating compliance to auditors: The lack of comprehensive audit trails and data governance features makes demonstrating compliance to auditors extremely difficult.
- Potential for hefty fines and legal repercussions: Non-compliance with data privacy and security regulations can result in significant fines and legal action.
- Reputational damage due to non-compliance: Data breaches and non-compliance can severely damage a company's reputation and erode customer trust.
Limited Scalability and Flexibility: A Stifling Environment for AI
Outdated business apps often lack the scalability and flexibility needed to support the demands of AI, creating further limitations.
Inability to Handle Increasing Data Volumes: Bottlenecks and Slowdowns
Legacy systems struggle to handle the massive data volumes required for effective AI training and operation, resulting in significant performance bottlenecks.
- System slowdowns and performance bottlenecks: As data volumes increase, outdated systems experience slowdowns and performance bottlenecks, hindering AI model training and deployment.
- Increased infrastructure costs to accommodate growth: Attempts to scale legacy systems to handle increasing data volumes lead to significantly higher infrastructure costs.
- Inability to scale AI models effectively: Limited scalability hinders the effective scaling of AI models, restricting the potential for broader application and improved performance.
- Limitations in processing speed and efficiency: The inherent limitations of legacy systems reduce processing speed and efficiency, impacting the effectiveness of AI implementations.
Lack of Adaptability to Changing Business Needs: Rigidity in a Dynamic World
Inflexible legacy systems hinder the rapid iteration and adjustment of AI models necessary for optimal performance.
- Difficulty in integrating new data sources and AI tools: Legacy systems often make it difficult to integrate new data sources and advanced AI tools, hindering innovation.
- Limited ability to adapt to evolving business requirements: The rigid nature of legacy systems makes it challenging to adapt to changing business requirements and market dynamics.
- Increased time and resources required for system upgrades: Upgrading legacy systems is often a complex and time-consuming process, requiring significant resources.
- Reduced agility and responsiveness to market changes: The lack of flexibility in legacy systems reduces a company's agility and responsiveness to market changes, putting them at a competitive disadvantage.
The Cost of Inaction: Missed Opportunities and Lost Revenue
Sticking with outdated business applications carries significant financial implications, including missed opportunities for AI-driven innovation and cost savings.
- Missed opportunities for automation and efficiency gains: Outdated systems prevent automation of numerous processes, leading to lost efficiency gains.
- Reduced profitability due to slower processing and higher error rates: Slow processing and higher error rates due to outdated systems directly impact profitability.
- Increased operational costs due to manual intervention and system maintenance: Manual intervention and frequent system maintenance significantly increase operational costs.
- Loss of competitive advantage due to slower innovation and responsiveness: Inability to leverage AI effectively puts a company at a significant competitive disadvantage.
Conclusion
Outdated business applications are not just inefficient; they actively obstruct your path towards a successful AI implementation. Addressing these legacy systems is crucial for unlocking the full potential of AI and achieving a competitive edge. By upgrading to modern, scalable, and secure applications, you can eliminate data silos, enhance data security, and gain the flexibility needed to effectively leverage AI. Don't let outdated business apps obscure your AI vision – take action today and embrace a future driven by intelligent automation. Modernize your business applications and unlock the power of AI.
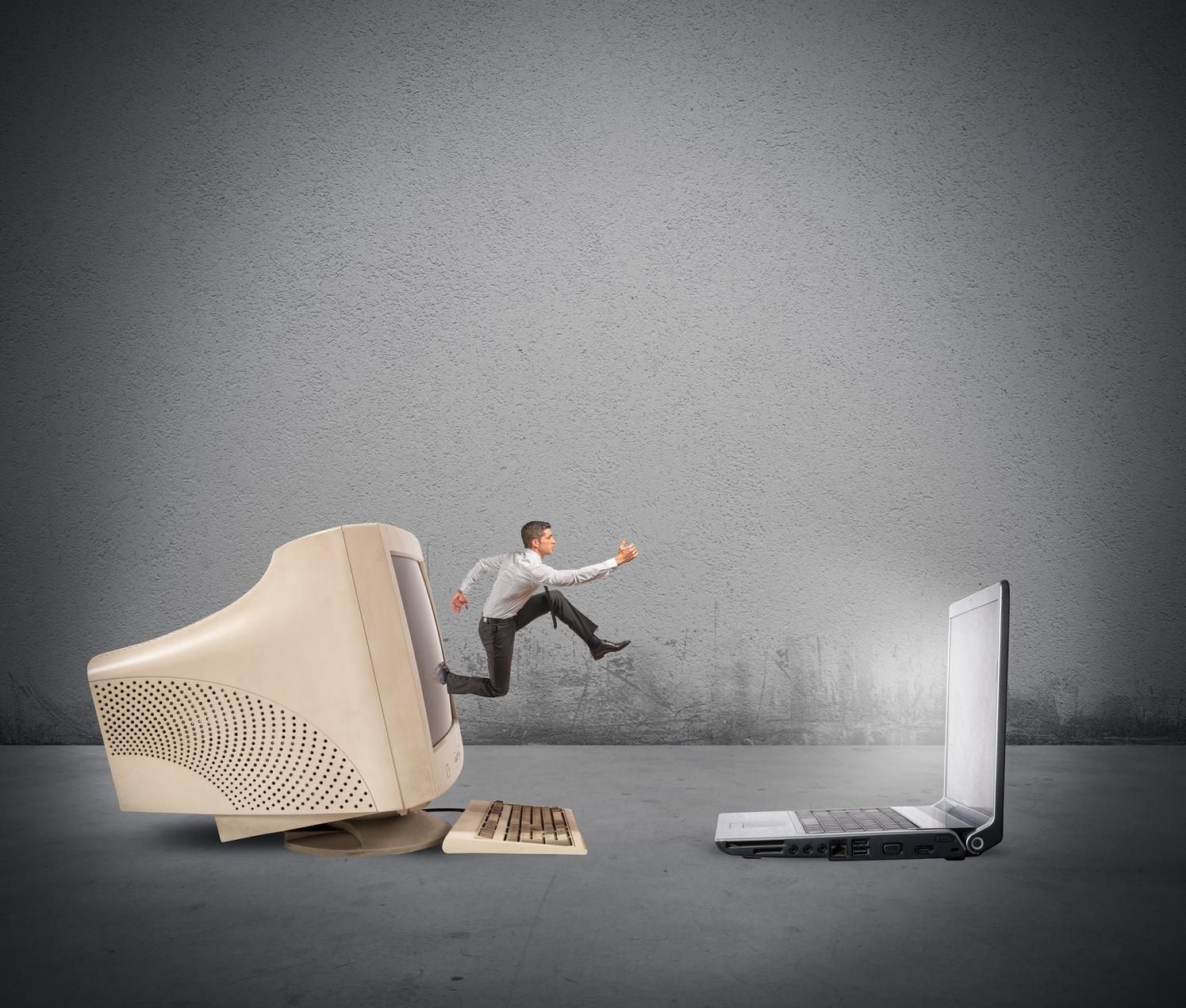
Featured Posts
-
44 Year Old Channing Tatum Reportedly Dating 25 Year Old Inka Williams
Apr 30, 2025 -
Kynyda Ke Ayndh Eam Antkhabat Tmam Tr Antzamat Mkml
Apr 30, 2025 -
Yankees Salvage Series Finale Rodons Dominant Performance Against Guardians
Apr 30, 2025 -
La Flaminia Sale In Classifica Dal Quinto Al Secondo Posto
Apr 30, 2025 -
Channing Tatum 44 And Inka Williams 25 Publicly Display Affection
Apr 30, 2025