Outdated Software Hindering Your AI Strategy?
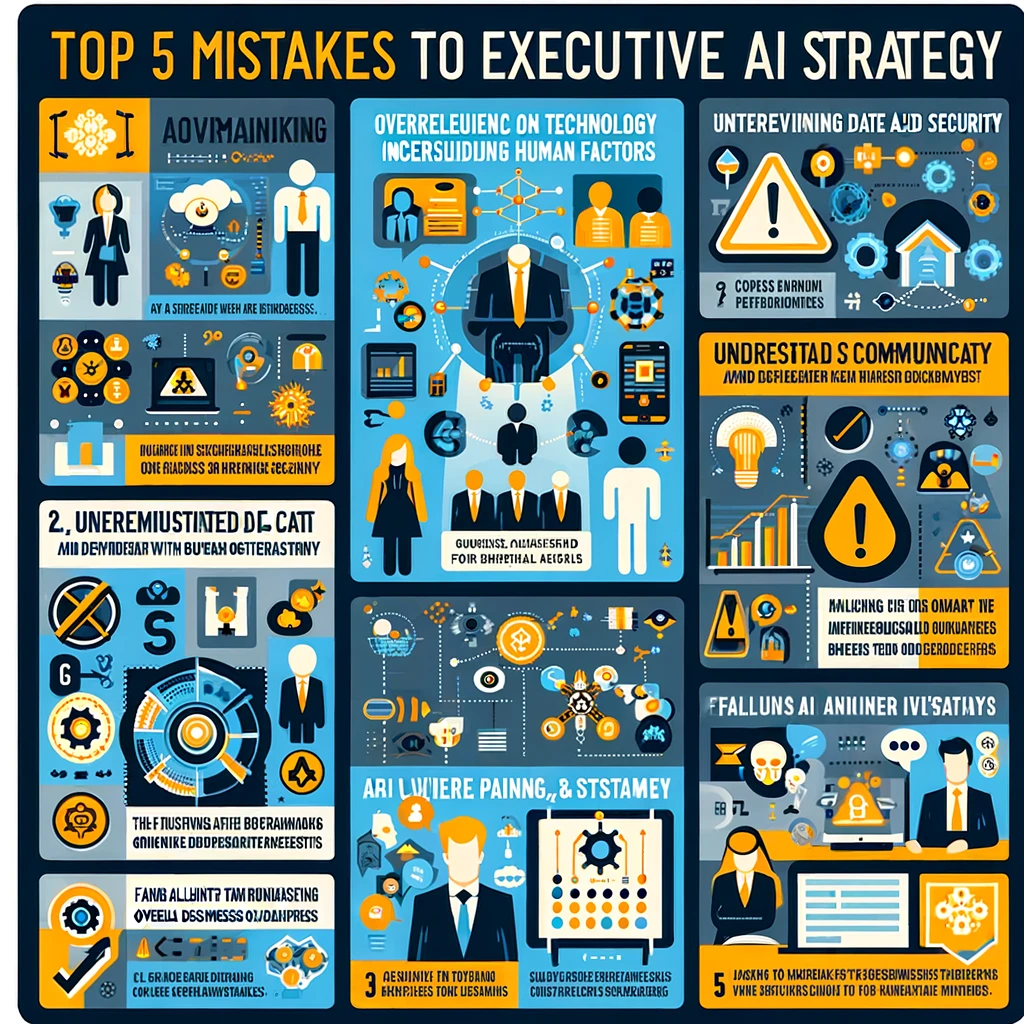
Table of Contents
Incompatibility with Modern AI Tools and Frameworks
Outdated software creates a significant barrier to effective AI implementation. The incompatibility between legacy systems and modern AI/ML tools presents numerous challenges.
Data Integration Challenges
Older systems often lack the Application Programming Interfaces (APIs) and data structures necessary for seamless integration with modern AI/ML platforms like TensorFlow and PyTorch. This leads to significant data integration challenges.
- Data silos: Data is scattered across different, incompatible systems, making it difficult to consolidate and analyze.
- Lack of real-time data streaming: Real-time data is crucial for many AI applications, but older systems often struggle to provide it.
- Difficulty in data cleaning and preprocessing: Preparing data for AI models is time-consuming, and outdated systems lack the tools to automate this process efficiently.
For example, imagine a company using an old CRM system that doesn't easily export data. Training a customer churn prediction model becomes incredibly difficult, hindering the ability to proactively address customer attrition.
Scalability Issues
Legacy software often struggles to handle the massive datasets required for effective AI training and deployment.
- Insufficient processing power: Older hardware may not be powerful enough to handle the computational demands of modern AI models.
- Memory constraints: Limited memory can restrict the size of datasets that can be processed, affecting model accuracy.
- Slow database queries: Inefficient database systems can significantly slow down the training and deployment process.
For instance, attempting to train a complex deep learning model on a legacy database system with slow query times will lead to unacceptably long training durations, hindering progress and increasing costs.
Security Risks
Outdated software presents increased security vulnerabilities, compromising sensitive data used in AI applications.
- Lack of security patches: Older systems often lack the latest security updates, leaving them vulnerable to cyberattacks.
- Outdated encryption protocols: Weak encryption can expose sensitive data to unauthorized access.
- Vulnerability to known exploits: Legacy systems are often susceptible to known vulnerabilities that can be exploited by malicious actors.
Using an outdated ERP system to store and process sensitive customer data for an AI-powered recommendation engine poses significant security risks, potentially leading to data breaches and reputational damage.
Lack of Necessary Features for AI Development
Beyond incompatibility, outdated software often lacks the fundamental features necessary for successful AI development.
Limited Automation Capabilities
Older systems may lack the automation features essential for efficient AI workflows.
- Manual data entry: Manual data entry is time-consuming, error-prone, and inefficient.
- Lack of automated workflows for model training and deployment: Automating these processes is vital for scaling AI initiatives.
Manually preparing data for model training is significantly less efficient than using automated pipelines which are commonly available in modern systems. This manual work increases costs and slows down development.
Absence of AI-Specific Functionality
Many legacy systems lack built-in support for crucial AI functionalities.
- Lack of model versioning: Managing multiple versions of AI models can become challenging without proper version control.
- Difficulty in tracking model performance: Monitoring and evaluating model performance is essential but difficult with limited tools.
- Lack of explainability tools: Understanding how AI models arrive at their predictions is crucial for trust and accountability.
Deploying and managing an AI model without a dedicated platform and integrated monitoring tools is incredibly challenging. It requires considerable manual effort and increases the risk of errors.
Difficulty in Integrating with Cloud Services
Older systems may struggle to integrate with cloud-based AI services, limiting scalability and cost-effectiveness.
- Compatibility issues with cloud APIs: Seamless integration with cloud services is vital for accessing powerful AI tools and infrastructure.
- Lack of cloud-native features: Cloud-native features improve scalability, efficiency, and cost-effectiveness.
- Difficulties in migrating data to the cloud: Migrating legacy data can be a complex and costly process.
Migrating legacy data to a cloud platform for AI training can be a significant undertaking, requiring considerable time and resources. This further delays AI project implementation.
The Cost of Inaction: Missed Opportunities and Financial Losses
Sticking with outdated software carries significant financial risks. The cost of inaction can manifest in several ways:
- Lost revenue: Inefficient processes and delayed product launches due to technological limitations directly impact revenue.
- Increased operational costs: Manual workarounds and inefficient workflows increase operational expenses.
- Competitive disadvantages: Competitors leveraging advanced AI capabilities gain a significant market advantage.
Studies show that companies with outdated systems experience a 20-30% reduction in operational efficiency and a 10-15% loss in potential revenue compared to those utilizing modern, AI-ready infrastructure.
Conclusion
Outdated software presents significant challenges to successful AI implementation. Incompatibility with modern tools, lack of essential features, and increased security risks lead to significant financial losses and missed opportunities. Don't let outdated software hinder your AI strategy. Legacy systems hindering AI progress are a major concern for businesses looking to stay competitive. Begin your modernization journey today by evaluating your current systems and exploring upgrade options. Modernizing software for AI success is a critical investment for long-term growth and competitiveness in the AI-driven landscape.
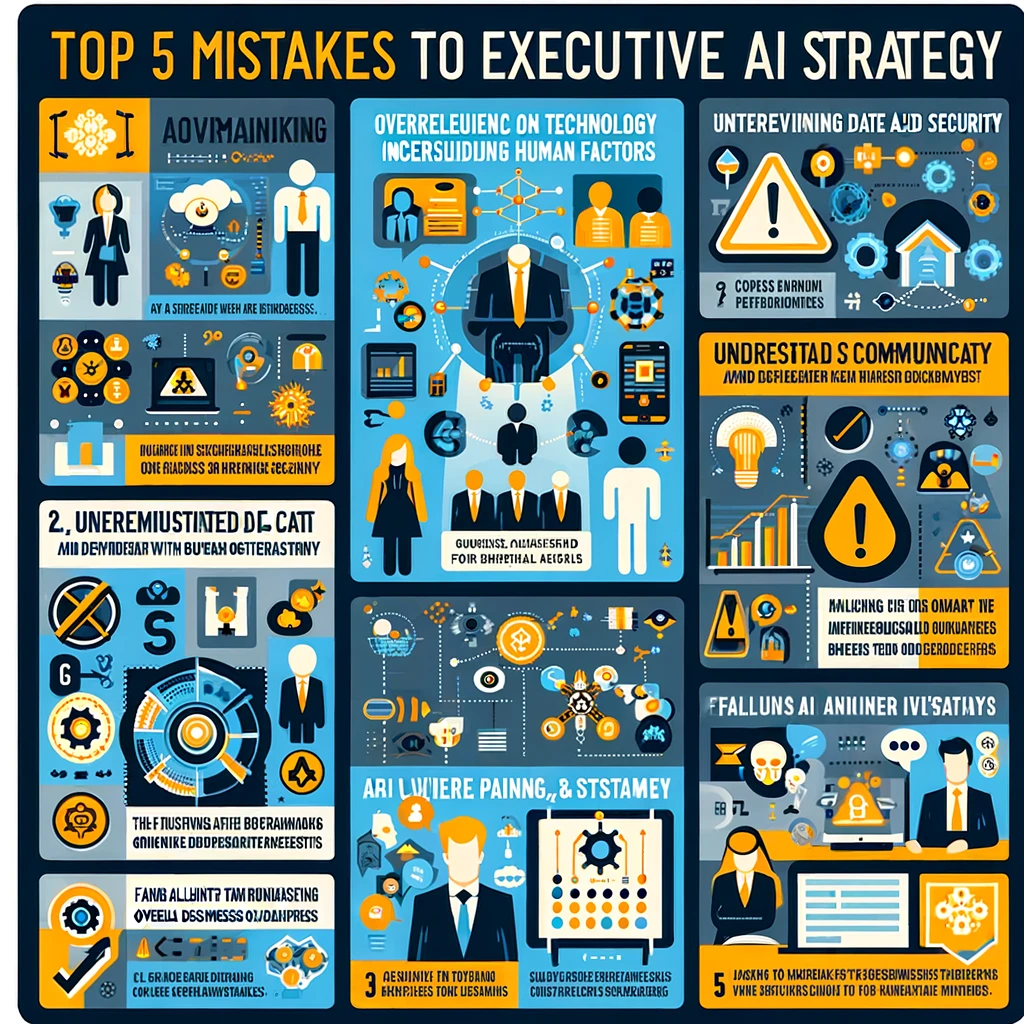
Featured Posts
-
Episima I Pari Tha Agonistei Ksana Stin Euroleague Tin Epomeni Sezon
Apr 30, 2025 -
Kentucky Storm Damage Assessments Delays And Reasons
Apr 30, 2025 -
The March Dance Roster Tracking Director And Dancer Appointments
Apr 30, 2025 -
Pacers Vs Cavaliers Complete Game Schedule Viewing Guide And Predictions
Apr 30, 2025 -
Who Are Remember Monday The Uks Eurovision 2025 Hopefuls
Apr 30, 2025