Using Social Media To Predict Economic Downturns: A Data-Driven Approach
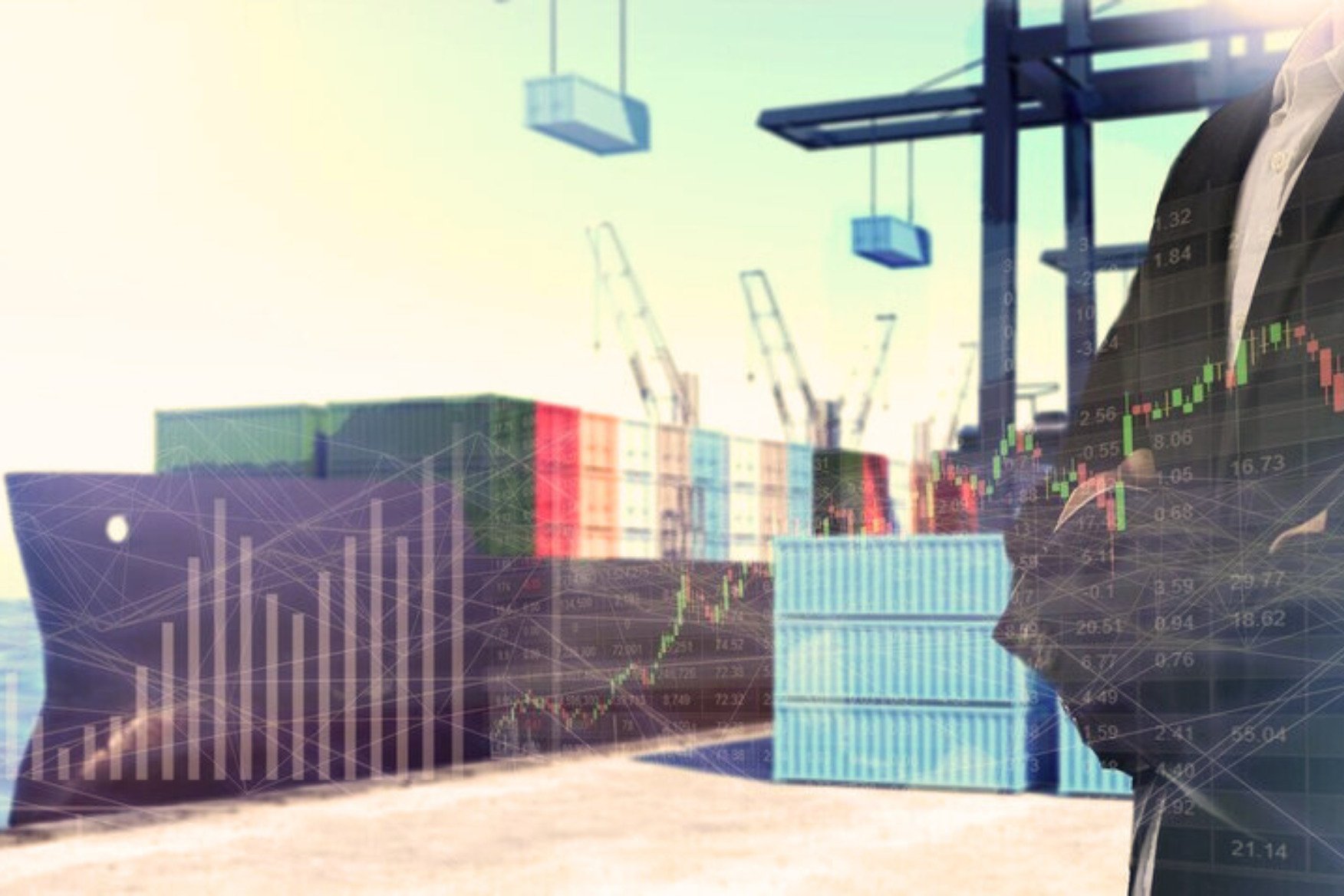
Table of Contents
Identifying Early Warning Signs on Social Media
Social media platforms offer a real-time pulse on public sentiment, consumer behavior, and employment trends – all vital indicators of economic health. By analyzing this data, we can identify early warning signs of potential downturns.
Sentiment Analysis of Social Media Conversations
Sentiment analysis, powered by Natural Language Processing (NLP), allows us to gauge public opinion towards the economy. Algorithms analyze textual data from platforms like Twitter, Facebook, and Reddit, identifying keywords and phrases reflecting economic anxiety. For example, a surge in posts containing phrases like "job loss," "layoffs," "financial crisis," or "economic recession" could signal growing unease.
- Specific examples of sentiment analysis tools: VADER (Valence Aware Dictionary and sEntiment Reasoner), TextBlob, and various cloud-based NLP APIs.
- Applications: These tools can track the sentiment surrounding specific industries, economic policies, or even individual companies, providing granular insights into potential vulnerabilities.
Tracking Consumer Spending Trends via Social Media
Social media provides a window into consumer behavior. By monitoring hashtags and keywords related to shopping (#sale, #discount, #deals), spending (#shopping spree, #online shopping), and even travel plans, we can identify shifts in spending habits. Location data further refines this analysis, revealing regional economic variations.
- Examples of social media data that reflects consumer spending: Posts about sales events, restaurant reviews mentioning price increases, discussions of travel plans (indicating discretionary spending), and posts about purchasing high-value items.
- A decrease in mentions of these activities could indicate reduced consumer confidence and potential economic slowdown.
Analyzing Social Media Data for Employment Trends
Platforms like LinkedIn and Glassdoor offer valuable data on employment trends. Analyzing job postings, discussions about unemployment, and employee reviews can help identify shifts in the job market. While social media data shouldn't replace official unemployment figures, it provides a valuable supplement, offering insights into sentiment and potential future trends.
- Examples of social media data points relevant to employment trends: Increased mentions of job searching, decreased number of job postings in specific sectors, negative employee reviews highlighting layoffs or salary cuts.
- A noticeable increase in negative sentiment related to employment can be a strong leading indicator.
Data Collection and Analysis Techniques
Effectively using social media to predict economic downturns requires a robust data collection and analysis strategy.
Choosing the Right Social Media Platforms
Each platform offers unique data. Twitter provides real-time sentiment, while Facebook offers demographic insights. LinkedIn focuses on professional networks, offering employment-related data. A multi-platform approach, combining data from various sources, is often most effective.
- Comparison table of relevant social media platforms and their data suitability:
Platform Strengths Weaknesses Twitter Real-time sentiment, broad reach Limited demographic data, potential for bias Facebook Demographic data, user groups, detailed posts Privacy concerns, data access limitations LinkedIn Professional network, employment-related data Limited reach outside the professional sphere Reddit Diverse communities, niche discussions Difficult to moderate, potential for misinformation
Utilizing Data Mining and Machine Learning
Machine learning algorithms are crucial for identifying patterns and correlations within large social media datasets. Techniques like sentiment analysis, time series forecasting, and anomaly detection can highlight significant shifts and predict future trends. Data cleaning and preprocessing are essential to ensure data accuracy and reliability.
- Examples of machine learning algorithms suitable for this task: Sentiment analysis models (e.g., Naive Bayes, Support Vector Machines), Recurrent Neural Networks (RNNs) for time series forecasting, anomaly detection algorithms.
The Importance of Data Visualization
Effective data visualization is essential for interpreting complex social media data. Dashboards and other interactive tools can present findings clearly and concisely, allowing for easier identification of key trends.
- Examples of effective visualization techniques for this type of data: Line charts showing sentiment trends over time, geographical maps highlighting regional variations in consumer spending, bar charts comparing job postings across different sectors.
Limitations and Challenges
While social media offers valuable insights, it's crucial to acknowledge the limitations and potential challenges.
Data Bias and Reliability
Social media data can be biased, reflecting the demographics and opinions of active users, potentially not representing the entire population accurately. It’s vital to cross-validate findings with other economic indicators to ensure reliability.
- Examples of biases: Geographical bias (overrepresentation of users from specific regions), demographic bias (overrepresentation of certain age groups or socioeconomic strata), platform-specific bias (each platform attracts a different user base).
Ethical Considerations
Using social media data for economic prediction raises ethical concerns regarding privacy and data security. Responsible data collection and analysis practices, respecting user privacy and adhering to data protection regulations, are paramount.
- Guidelines for responsible data collection and analysis: Obtain informed consent where necessary, anonymize data appropriately, ensure data security, and transparently communicate the purpose of data collection.
Conclusion
Using social media to predict economic downturns offers a powerful, data-driven approach to economic forecasting. By analyzing sentiment, consumer behavior, and employment trends reflected in social media conversations, combined with robust data analysis techniques, we can gain valuable insights into potential economic instability. However, it's crucial to be aware of data biases, ethical considerations, and to always validate findings with other economic indicators. Start leveraging the power of social media analytics to improve your understanding of economic trends and gain a competitive edge by effectively using social media to predict economic downturns.
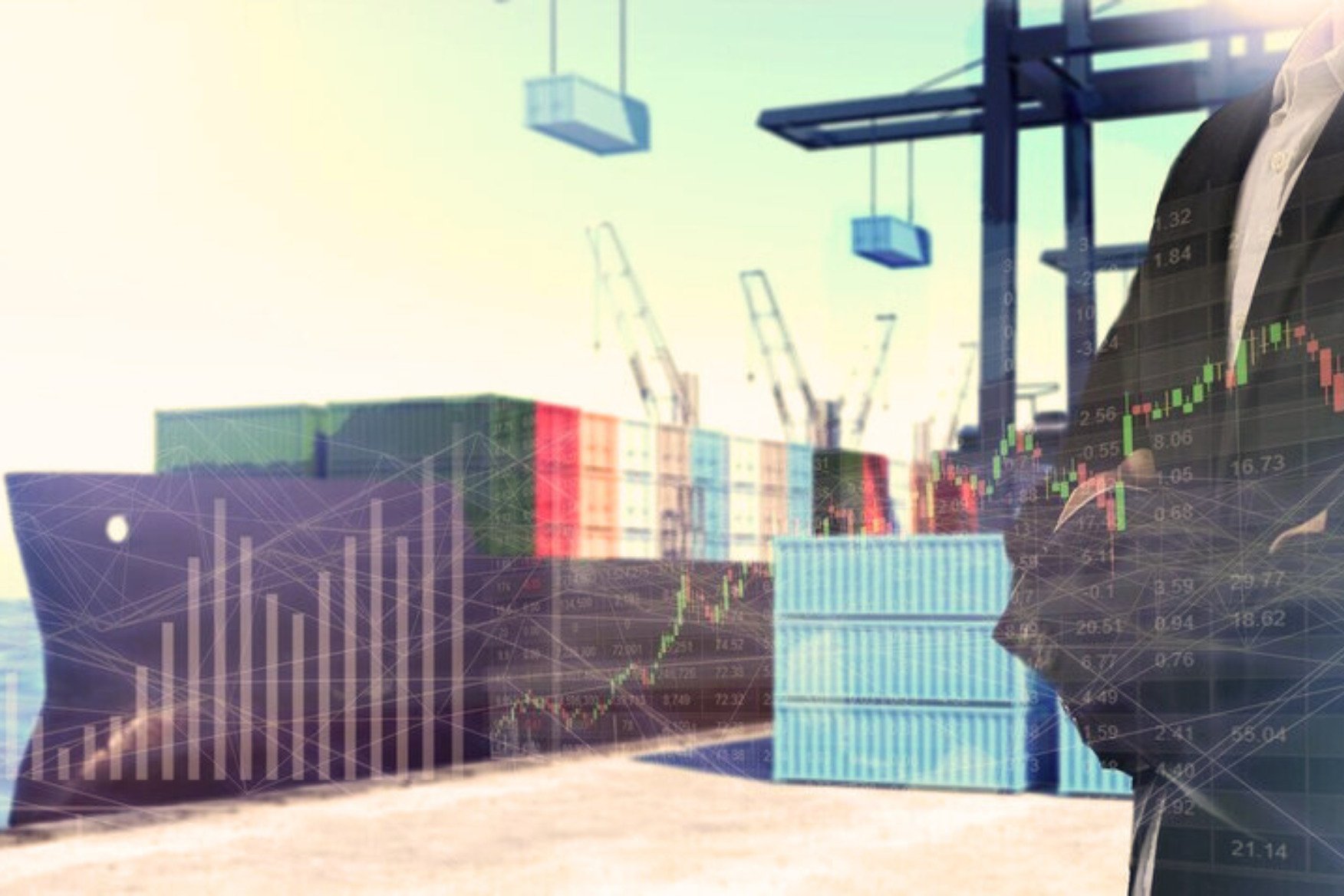
Featured Posts
-
Watch Gypsy Rose Life After Lockup Season 2 A Complete Guide
May 06, 2025 -
Kim Kardashian A Retrospective Of Her Best Hair And Makeup Styles
May 06, 2025 -
Gazze Skandali Trump Dansoezler Altin Elon Musk Ve Netanyahu Ayrintilari
May 06, 2025 -
Fortnite Update Sabrina Carpenter Dance Emotes Arrive
May 06, 2025 -
Strengthening Ties The Us Israel Azerbaijan Strategic Partnership And Regional Stability
May 06, 2025